Google AI Delivers Essential Savings with New Implicit Caching for Gemini Models
3 min read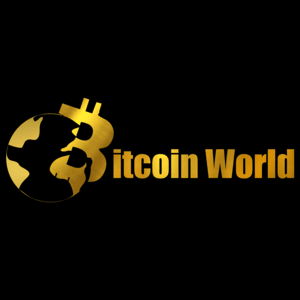
In the rapidly evolving world where AI intersects with blockchain and digital assets, managing infrastructure costs is crucial. Developers leveraging powerful AI models often face significant expenses. Google has recently launched a new feature in its Gemini API, called ‘implicit caching’, aiming to dramatically reduce these costs, which is welcome news for anyone building in the space. What is Google AI Implicit Caching? Google’s new ‘implicit caching’ is designed to make accessing its latest AI models , specifically Gemini 2.5 Pro and 2.5 Flash, significantly cheaper for third-party developers. This feature is a more automated approach to caching data that is frequently sent to the models. Caching is a standard technique in the AI industry. It involves storing and reusing data or computations that are accessed often. This reduces the need for the model to process the same information repeatedly, thereby cutting down on computing power requirements and, importantly, cost. Google claims that implicit caching can deliver substantial savings, potentially up to 75%, on what they term ‘repetitive context’ passed to the models via the Gemini API . This is particularly beneficial for applications where users frequently ask similar questions or where a common set of instructions or data is provided at the beginning of prompts. Implicit vs. Explicit: Why the Change? Before implicit caching, Google offered ‘explicit prompt caching’. This required developers to manually identify and define the prompts they used most frequently. While intended to provide cost savings, developers reported that explicit caching often involved considerable manual effort. Furthermore, some developers expressed dissatisfaction with its implementation for Gemini 2.5 Pro, citing unexpectedly high developer costs . Complaints regarding these costs reportedly increased recently, prompting the Gemini team to issue an apology and commit to making improvements. Implicit caching appears to be a direct response to this feedback. The key difference is automation. Implicit caching works automatically by default for Gemini 2.5 models. If a request sent through the Gemini API shares a common starting point or ‘prefix’ with a previous request that is stored in the cache, the system automatically applies the cost savings. According to Google’s developer documentation, the minimum prompt token count required to trigger implicit caching is 1,024 for Gemini 2.5 Flash and 2,048 for Gemini 2.5 Pro. A thousand tokens is roughly equivalent to 750 words. These minimums are not particularly high, suggesting that developers should be able to benefit from automatic savings without needing very long prompts. Achieving Maximum Savings with Implicit Caching While implicit caching is automatic, Google offers a tip for developers to maximize its effectiveness and ensure they see the promised 75% reduction in developer costs for cached hits. Google recommends structuring requests so that repetitive context (information that stays the same across multiple prompts, like instructions or background data) is placed at the beginning of the request. Context that changes from one request to the next (like the user’s specific question or query) should be appended towards the end. This increases the likelihood that the beginning of the prompt will match a cached prefix, triggering the automatic savings. Given the previous issues with cost expectations and explicit caching, some developers may approach these new claims with caution. Google has not yet provided third-party verification of the 75% savings figure. Therefore, the actual impact on developer costs will become clearer as early adopters share their experiences. Summary: Lowering the Barrier to Advanced AI Google’s introduction of implicit caching for its Gemini 2.5 AI models through the Gemini API is a significant development aimed squarely at reducing developer costs . By automating the caching process, Google is making it easier and potentially much cheaper for developers to leverage powerful frontier models, addressing previous criticisms regarding pricing and manual caching efforts. While developers should follow Google’s recommendations on prompt structure to optimize savings and observe real-world results, this feature represents a promising step towards making advanced Google AI more accessible and economically viable for a wider range of applications, including those potentially integrating with the cryptocurrency and blockchain ecosystem. To learn more about the latest AI models trends, explore our article on key developments shaping AI features.
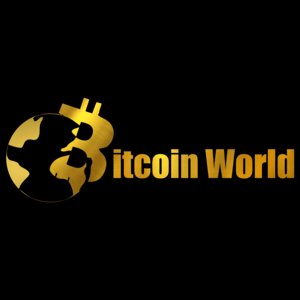
Source: Bitcoin World