DeepMind AlphaEvolve Unleashes Breakthrough in AI Math Problems
4 min read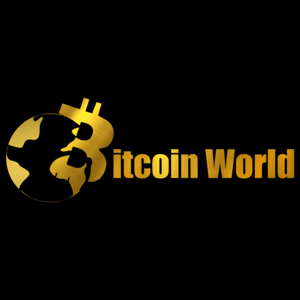
BitcoinWorld DeepMind AlphaEvolve Unleashes Breakthrough in AI Math Problems In the rapidly evolving world of artificial intelligence, breakthroughs are constantly reshaping what’s possible. For those following the intersection of tech and digital assets, understanding these advancements is key. Google’s cutting-edge AI research lab, DeepMind, has unveiled its latest innovation: a system designed to tackle complex math and science challenges. Let’s dive into what DeepMind AlphaEvolve is and why it matters. What is DeepMind AlphaEvolve? DeepMind AlphaEvolve is a new AI system specifically engineered to solve problems that have “machine-gradable” solutions. This means it excels at tasks where the correctness of an answer can be automatically verified, typically through formulas, code execution, or logical checks. Unlike general-purpose AI models, AlphaEvolve focuses on domains where precision and verifiability are paramount, such as mathematics, computer science, and system optimization. DeepMind states that AlphaEvolve utilizes “state-of-the-art” models, specifically leveraging the power of their Gemini models, to achieve its capabilities. This foundation is claimed to make it significantly more potent than previous AI systems attempting similar tasks. Tackling the Challenge of AI Hallucination One of the most significant hurdles in developing reliable AI systems is the tendency for models to “hallucinate.” Due to their probabilistic nature, AI models can sometimes confidently generate incorrect or nonsensical information. This issue is particularly challenging in complex fields where accuracy is critical. DeepMind AlphaEvolve introduces a clever mechanism to combat this problem: an automatic evaluation system. Here’s how it works: The system generates a pool of potential answers to a given problem. It then uses internal models or predefined criteria to critique these potential solutions. Finally, it automatically evaluates and scores the answers based on their accuracy using the provided machine-gradable assessment mechanism. This iterative process of generation, critique, and evaluation allows AlphaEvolve to significantly reduce the occurrence of hallucinations compared to systems that simply generate a single output. Solving AI Math Problems and Beyond AlphaEvolve is specifically designed for domain experts who can provide not just the problem but also a method for verifying the solution. Users prompt the system with a problem, which can include details like instructions, equations, code snippets, or relevant literature. Crucially, they must also provide a formula or mechanism for automatically assessing the system’s answers. Because it relies on this self-evaluation capability, AlphaEvolve is best suited for problems where the solution’s correctness can be programmatically checked. This includes areas like: Solving complex mathematical equations. Optimizing algorithms and code. Improving the efficiency of technical systems. It’s important to note a key limitation: AlphaEvolve can primarily describe solutions as algorithms or numerical outputs. This makes it less suitable for problems that require non-numerical or descriptive answers. Real-World AI Optimization at Google To demonstrate AlphaEvolve’s capabilities, DeepMind conducted benchmarks using both theoretical problems and practical, real-world challenges within Google’s infrastructure. In tests involving a curated set of around 50 math problems spanning various branches, AlphaEvolve achieved impressive results: It rediscovered the best-known answers to problems 75% of the time. It uncovered improved solutions in 20% of cases. Beyond theoretical math, DeepMind also evaluated AlphaEvolve on practical problems crucial to Google’s operations, focusing on AI Optimization . The system generated an algorithm that continuously recovers, on average, 0.7% of Google’s worldwide compute resources. While this might sound small, for a company the size of Google, this represents a significant amount of processing power. It also suggested an optimization that reduced the overall time required to train Google’s powerful Gemini models by 1%. Training large AI models is incredibly time-consuming and resource-intensive, so even a 1% reduction is a substantial efficiency gain. These examples highlight AlphaEvolve’s potential to drive tangible improvements in the efficiency of large-scale technical systems. Google DeepMind AI: A Focus on Efficiency DeepMind emphasizes that AlphaEvolve’s primary value lies not necessarily in making entirely novel, breakthrough scientific discoveries, but in significantly boosting efficiency and freeing up human experts. While AlphaEvolve might identify optimizations that have been previously flagged by other tools or human analysis, its ability to do so automatically and rapidly is its core strength. The system can automate the tedious process of searching for optimal solutions in complex systems, allowing engineers and researchers to focus their time and expertise on higher-level challenges and creative work. This aligns with a broader trend in AI development: building tools that augment human capabilities and improve productivity. Looking Ahead DeepMind is currently developing a user interface to make AlphaEvolve more accessible. They plan to launch an early access program for selected academics, gathering feedback before a potential broader rollout. This phased approach allows for refinement and ensures the tool is robust and useful for its intended users. While AlphaEvolve has limitations, particularly in the types of problems it can handle, its success in tackling complex AI Math Problems and achieving significant optimization gains within Google’s infrastructure demonstrates a promising path forward for developing more reliable and efficient AI problem-solvers. The system’s unique approach to mitigating AI Hallucination through automated evaluation is a notable technical achievement. In conclusion, DeepMind AlphaEvolve represents a significant step in building AI systems that can reliably solve complex, verifiable problems. By focusing on machine-gradable solutions and employing an innovative self-evaluation mechanism, it offers a powerful tool for optimization and research, promising to enhance the efficiency of AI development and technical systems at scale. To learn more about the latest AI trends, explore our article on key developments shaping AI features. This post DeepMind AlphaEvolve Unleashes Breakthrough in AI Math Problems first appeared on BitcoinWorld and is written by Editorial Team
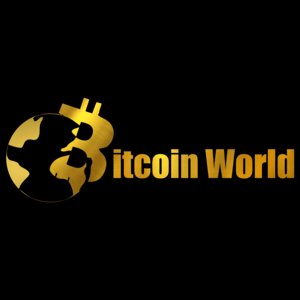
Source: Bitcoin World