Reasoning AI Models Face Potential Limit on Future Gains, Analysis Finds
3 min read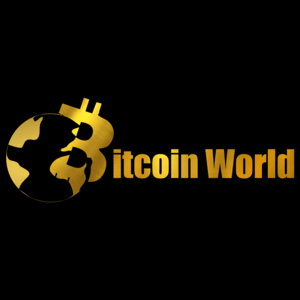
In the fast-evolving world of technology, where AI breakthroughs often dominate headlines, a new analysis introduces a note of caution. For those tracking the intersection of tech innovation and its broader market implications, including the crypto space which often leverages cutting-edge AI, understanding the nuances of AI progress is crucial. A recent report from Epoch AI, a non-profit AI research institute, suggests that the rapid improvements seen in certain advanced reasoning AI models might not be sustainable at their current pace. Are Reasoning AI Models Hitting a Wall? The core finding from Epoch AI indicates that the AI industry may struggle to achieve massive performance gains from reasoning AI models for much longer. The analysis points to a potential slowdown in progress as soon as within a year. This comes after a period where models like OpenAI’s o3 have shown significant leaps, particularly on benchmarks involving complex tasks like math and programming. These models excel by applying more computational power to problems, though this comes at the cost of increased processing time compared to conventional models. Understanding Reasoning Models and Reinforcement Learning How are these advanced models developed? The process typically involves two main stages: Initial Training: A conventional model is first trained on vast datasets. Reinforcement Learning: A technique is then applied where the model receives feedback on its attempts to solve difficult problems. This feedback loop helps refine its reasoning abilities. Epoch AI notes that while frontier labs like OpenAI have scaled up computing for the initial training, the reinforcement learning stage hasn’t historically received the same massive allocation. However, this is changing. OpenAI reportedly used about 10 times more computing power for o3 compared to its predecessor, o1, with Epoch speculating much of this went into reinforcement learning. OpenAI researchers have also indicated future plans to prioritize reinforcement learning with even more computational resources. The Epoch AI Analysis: Scaling Limits Despite the increased focus on reinforcement learning, the Epoch AI analysis suggests there’s an upper bound to how much computing can effectively be applied to this stage. Josh You, the author of the analysis, highlights the difference in current scaling rates: Performance gains from standard AI model training are currently quadrupling annually. Performance gains from reinforcement learning are growing tenfold every 3-5 months. You predicts that the progress from reasoning training will likely converge with the overall frontier AI progress by 2026. The analysis acknowledges making assumptions and drawing on public statements from AI executives, but it strongly argues that scaling reasoning models faces challenges beyond just computing power. Challenges for the AI Industry The possibility that reasoning AI models may reach a limit in the near future is a significant concern for the AI industry . Companies have invested enormous resources into developing these complex models. Beyond the computational scaling issues highlighted by Epoch AI, other factors could impede progress, including high overhead costs associated with research and development. You writes, “If there’s a persistent overhead cost required for research, reasoning models might not scale as far as expected.” He emphasizes the importance of tracking rapid compute scaling closely, as it’s a potentially very important ingredient for progress in these models. Furthermore, studies have already pointed out significant flaws in these models, despite their power and expense, such as a tendency to “hallucinate” or produce incorrect information more often than some simpler models. Conclusion: What This Means for Future AI Progress The Epoch AI analysis provides a valuable perspective on the potential trajectory of AI progress , particularly concerning advanced reasoning capabilities. While breakthroughs continue to happen, understanding potential bottlenecks, whether computational or research-related, is vital for setting realistic expectations and directing future efforts within the AI industry . The rapid gains from reinforcement learning that have fueled recent progress may slow as they hit scaling limits, potentially requiring new fundamental approaches to continue the pace of innovation in reasoning AI models . To learn more about the latest AI market trends, explore our article on key developments shaping AI features.
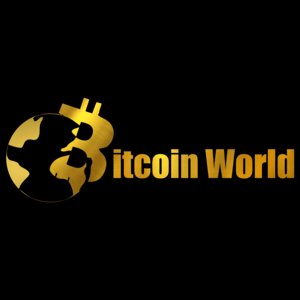
Source: Bitcoin World